Know The Difference Between Artificial Intelligence And Machine Learning
4.9 out of 5 based on 9321 votesLast updated on 9th Nov 2023 6.4K Views
- Bookmark
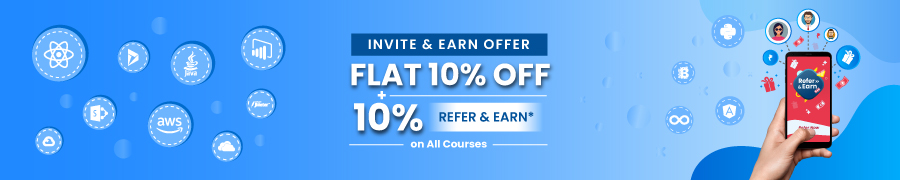
AI is broader, simulating human intelligence, while ML is a subset, focusing on learning from data for specific tasks.
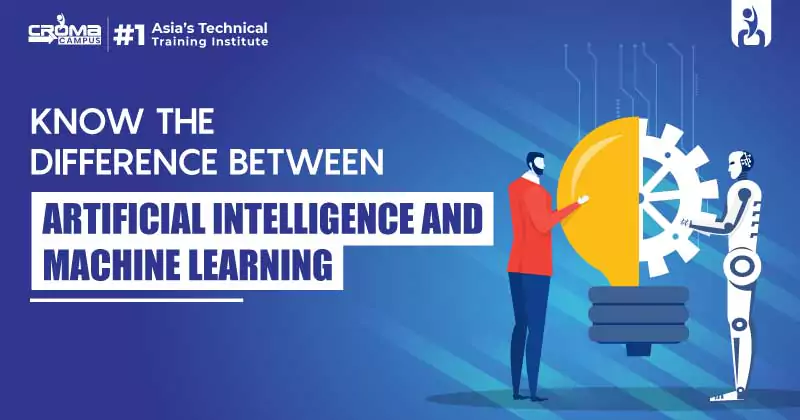
This blog mainly covers the difference between Artificial Intelligence (AI) and Machine Learning (ML), explaining various AI categories, the concept of AI & ML, and types of machine learning.
- Overview
- What is Artificial Intelligence?
- AI Categories
- What is Machine Learning?
- Types of Machine Learning
- Difference between Artificial intelligence and machine learning
- Key Takeaways
Overview:
Artificial intelligence (AI) and Machine Learning (ML) are the two most celebrated domains in the computer science world. They are used to build intelligence systems that can be further utilized by different industries to increase their productivity.
People are usually confused among the two domains but they are slightly different and can be used for different purposes. AI can be taken as clever technology that works similarly to humans. Machine Learning is a branch of AI. In this blog, we will understand, what is AI, what is ML and how are they different from each other.
Artificial Intelligence – Know About AI & Its Categories
AI is a powerful technology helping humans to create super machines that can work similarly to them. Soon, there will come a time when everything will be AI-enabled from devices to smartphones and smartwatches.
AI is further divided into three types based on its capabilities: Weak AI, General AI, and Strong AI. Currently, we mainly work with Weak AI and General AI. The future holds the promise of Strong AI, which is expected to be more intelligent than humans.
You May Also Read: Data Science Qualification
Artificial Intelligence - AI Categories
- Weak AI (Artificial Narrow Intelligence):
- Weak AI is like a specialist. It's smart at one specific thing.
- Imagine a computer program that's good at playing chess, but it can't do anything else. That's weak AI.
- It's narrow in its abilities and can't think or understand like a human.
- General AI (Artificial General Intelligence or AGI):
- General AI is like a human brain with incredible capabilities.
- Think of it as a computer or robot that can play chess, cook, drive a car, and even write a poem.
- It's like a multi-talented human in the form of a machine.
- Strong AI (Artificial Superintelligence):
- Strong AI is like a super-smart robot that's even smarter than the smartest humans.
- It can understand, learn, and solve problems just like humans, but it can do it much, much better.
- This level of AI is still in the future, and we're not there yet.
So, Weak AI is specialized, General AI is versatile like a human, and Strong AI is super-smart and beyond what we have today.
What is Machine Learning?
Machine Learning is a branch of Artificial Intelligence that empowers computers to learn without explicit programming. This concept, introduced by computer scientist Arthur Samuel in 1959, is all about teaching machines to improve their performance based on past experiences. It's like enabling a computer to acquire skills and make predictions for future tasks. Machine Learning aims to reach a level of performance at least comparable to human abilities.
In Technical Terms:
According to Tom M. Mitchell in 1997, Machine Learning occurs when a computer program learns from experience (E) in the context of tasks (T) and performance measures (P). The system's performance in task T, as measured by P, should improve with the accumulation of experience E.
Example: Handwriting Recognition
Consider a handwriting recognition problem where the machine is trained to recognize and classify handwritten words within images. In this scenario:
- Task (T): Recognizing and classifying handwritten words within images.
- Performance Measure (P): This is quantified by the percentage of words correctly classified, also known as accuracy.
- Training Experience (E): Comprises a data set of handwritten words with their given classifications.
For this particular activity, the machine gains knowledge from the given data set, which is simply an assortment of numerous samples. Every example has a collection of characteristics. It gets more adept at identifying and categorizing handwritten words within photographs the more examples it sees.
Machine learning is a significant tool in the field of technology and problem-solving because, in essence, it is the art of allowing computers to get better at tasks by learning from their experiences.
You May Also Read:
Learn About Machine Learning Fundamentals
Artificial Intelligence Certification Cost
Machine Learning and Deep Learning
Machine Learning Interview Questions and Answers
Machine Learning Categories
1. Supervised Learning:
- In supervised learning, we give the machine examples with labels or target values. This helps the machine understand how the features relate to the desired outcomes.
- Classification: Here, the machine predicts categories or labels. For example, it can predict if a stock's price will go up or down or classify news articles into topics like politics or leisure.
- Regression: In this, the machine predicts continuous values. For instance, it can forecast how much a new product will sell or estimate a job's salary based on the job description.
2. Unsupervised Learning:
Unsupervised learning is for when data doesn't have labels. To uncover hidden patterns in the data is the aim. Clustering is the process of assembling comparable examples into groups.
3. Reinforcement Learning:
Reinforcement learning is about teaching machines to achieve goals. They learn to make the best decisions in a given situation to maximize performance. Like rewards, feedback helps machines decide what to do next. It can, for instance, score the most points throughout several moves in a game.
Your can find top machine learning courses here
Artificial Intelligence and Machine Learning
Here's the information presented in a table format for easier comparison:
You May Also Read: Best Machine Learning Certification Program
Key Takeaways:
- Artificial Intelligence (AI) is about making machines that can simulate human behavior, while Machine Learning (ML) is a subset of AI that focuses on machines learning from data without explicit programming.
- AI is divided into three types: Weak AI (specialized), General AI (versatile), and Strong AI (super-smart, beyond humans). ML mainly works with Weak and General AI.
- Machine Learning aims to make computers learn from past experiences, improve their performance, and reach human-level abilities. It involves tasks like classification and regression.
- AI has a broader scope, while ML is more focused. AI aims to create intelligent systems for various complex tasks, while ML trains machines for specific purposes.
- The main applications of AI include Siri, customer support, expert systems, games, and humanoid robots. ML is used for recommender systems, search algorithms, and friend tagging.
- AI can be categorized into Weak AI, General AI, and Strong AI based on its capabilities. ML can be categorized into Supervised, Unsupervised, and Reinforcement Learning.
- AI involves learning, reasoning, and self-correction, while ML focuses on learning and self-correction with new data.
- AI deals with structured, semi-structured, and unstructured data, whereas ML primarily deals with structured and semi-structured data.
Subscribe For Free Demo
Free Demo for Corporate & Online Trainings.
Your email address will not be published. Required fields are marked *
Course Features





