What is Machine Learning: Definitions, Variants, Tools, Applications, Recent Trends, And Beyond
5 out of 5 based on 47655 votesLast updated on 20th Dec 2023 563K Views
- Bookmark
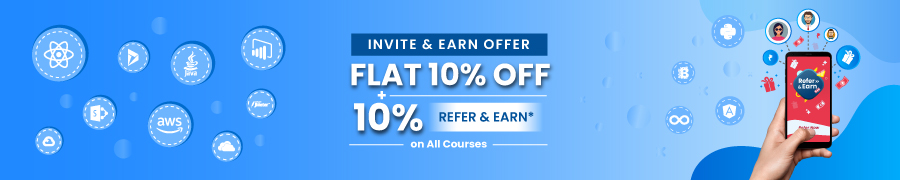
Machine Learning: A field of AI enabling computers to learn from data, improve performance, and make predictions without explicit programming.
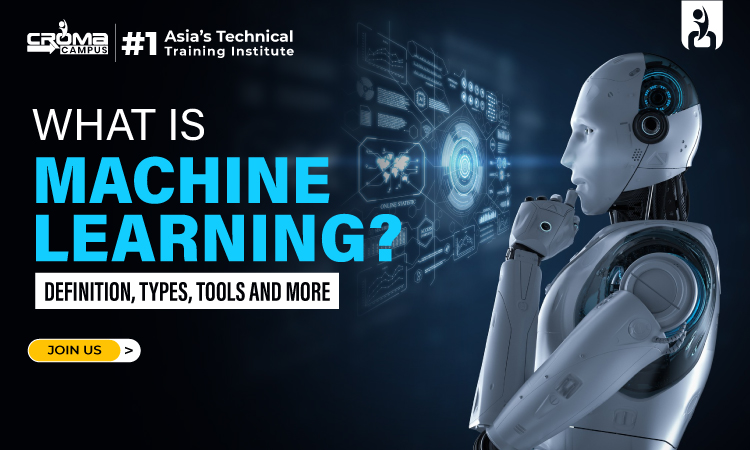
TOC:
Discover comprehensive insights into machine learning for 2024: Explore its types, applications, career paths, and the initial steps to enter this industry.
- Overview
- What is Machine Learning?
- Machine Learning vs AI vs Deep Learning
- Significance of Machine Learning
- Machine Learning Workflow
- Types of Machine Learning
- Machine Learning Impact
- Machine Learning Applications
- Machine Learning Tools
- Top Machine Learning Careers
- How to start a career in Machine Learning
- Final Thoughts
Overview:
In today's age of digital evolution, grasping the driving forces behind innovation isn't just advantageous—it is essential. Machine learning stands prominently among these transformative technologies. This article explains machine learning simply. It's for beginners and fans alike. We will cover what it is, types, uses, and tools. Additionally, we will talk about jobs and how to start a career in this cool field.
What is Machine Learning?
Machine learning, a part of artificial intelligence (AI), involves teaching computers to learn and improve at tasks using data rather than explicit programming. Instead of giving direct instructions, it learns from examples. For instance, to recognize cats, it studies many cat pictures to understand common features.
- Machine learning relies on algorithms that improve with more data.
- It doesn't need explicit programming for every task; it learns from examples.
- For instance, it helps in recognizing patterns like identifying objects in images or making predictions.
In essence, machine learning's ability to learn from data and adapt over time drives advancements in technologies like voice assistants, self-driving cars, and predictive analytics.
Understanding the Differences: Machine Learning, AI, and Deep Learning
The terms machine learning, artificial intelligence (AI), and deep learning often get tangled up, but they represent distinct concepts. AI encompasses programs designed to act intelligently, mirroring human cognition through specific algorithms. It emphasizes learning, reasoning, and self-correction to achieve optimal performance. AI can encompass machine learning-based systems or explicitly programmed ones.
Machine learning, a subset of AI, employs algorithms that learn from data to make predictions. It encompasses supervised learning, where patterns are learned from existing data, and unsupervised learning, where general patterns are discovered. ML models predict numerical values, categorize events, and cluster data based on similarities within the dataset.
In contrast, deep learning is a branch of machine learning centered on complex, multi-layered artificial neural networks (ANNs) inspired by the human brain's structure. Unlike traditional ML, deep learning models are nonlinear, intricate, and hierarchical. They excel at learning from vast datasets and yield remarkably accurate outcomes. Applications include language translation, image recognition, and personalized medicine.
Factors | Machine Learning | Artificial Intelligence (AI) | Deep Learning |
Focus | Learns from data | Emulates human intelligence | Multi-layered neural networks |
Learning Approach | Data-driven predictions | Learning, reasoning, self-correction | Hierarchical neural networks |
Techniques | Supervised and unsupervised learning | Diverse algorithms | Complex neural networks |
Application | Predictions, categorization, clustering | Various intelligent tasks | Image recognition, language translation, medicine |
Understanding these distinctions helps navigate their applications and functionalities within technology and research landscapes.
You May Also Read: Data Science and Machine Learning
Significance of Machine Learning:
In today's digital era, data holds the same significance as oil did in the past century. Machine learning stands as the driving force behind this data-centric world, and its pivotal role is indispensable. This significance is evident in the industry's expected surge, as forecasted by the US Bureau of Labor Statistics, estimating a 21% job growth from 2021 to 2031.
Here's why machine learning is so crucial in our modern landscape:
- Data Handling: With an overflow of digital data from sources like social media and sensors, traditional analysis methods fall short. Machine learning algorithms excel in processing vast data volumes, unveiling hidden patterns, and offering insights crucial for decision-making.
- Fueling Innovation: Machine learning sparks innovation and efficiency across diverse sectors:
- Healthcare: Predicting outbreaks, personalizing treatments, and enhancing medical imaging accuracy.
- Finance: Utilized in credit scoring, algorithmic trading, and fraud detection.
- Retail: Enhancing recommendation systems, supply chains, and customer service.
- Its applications span agriculture, education, and entertainment.
- Automation Catalyst: Crucial for automation, machine learning algorithms, through continuous learning from data, take on tasks once manual. This shift liberates humans to focus on intricate, creative endeavors. This not only boosts efficiency but also fosters new avenues for innovation.
You May Also Read: Artificial Intelligence and Machine Learning
Machine Learning Workflow: Step by Step
Understanding how machine learning operates involves breaking down a sequence that turns raw data into valuable insights. Here's a beginner's guide to the workflow:
- Step 1: Data Collection Begin by gathering data, the lifeblood of machine learning, from various sources like databases, text, images, or the web. Organize it into a suitable format and ensure relevance to the problem at hand.
- Step 2: Data Preprocessing Clean, handle missing data, and normalize it to enhance quality and ensure accurate interpretation by your model. This step significantly impacts model accuracy.
- Step 3: Model Selection Choose a suitable machine learning model (e.g., linear regression, decision trees, neural networks) based on the data nature, problem complexity, and available resources.
- Step 4: Model Training Feed prepared data into the chosen model, enabling it to adjust parameters for better predictions. Avoid overfitting or underfitting during this phase.
- Step 5: Model Evaluation Assess model performance using metrics like accuracy, precision, recall, or mean squared error on new, unseen data. This step ensures reliability before deployment.
- Step 6: Hyperparameter Tuning Optimize model performance by adjusting hyperparameters, employing techniques like grid search or cross-validation for improved accuracy.
- Step 7: Predictions and Deployment Utilize the trained and optimized model to make predictions on new data. Integrate it into a production environment for real-time insights, often referred to as MLOps.
Each step plays a critical role in transforming data into actionable insights, contributing to the effectiveness of machine learning models.
Machine Learning Types:
Supervised Learning:
- Definition: Supervised learning involves training a model on a labeled dataset, where inputs and desired outputs are provided. The algorithm learns to map input data to the correct output by making predictions and adjusting its parameters based on the given labels.
- Example: If you're teaching a model to recognize cats and dogs, you provide it with labeled images—identifying which ones contain cats and which ones contain dogs. The algorithm learns the patterns that distinguish cats from dogs.
- Applications: Commonly used in classification and regression tasks, such as spam detection in emails, image classification, or predicting housing prices.
Unsupervised Learning:
- Definition: Unsupervised learning involves training a model on unlabeled data, where the algorithm explores the data's structure and identifies patterns or relationships without explicit guidance.
- Example: Clustering similar data points together without prior knowledge of their categories. For instance, grouping customers based on purchasing behavior without specific labels.
- Applications: Widely used in clustering, anomaly detection, and dimensionality reduction, such as customer segmentation, detecting unusual patterns in data, or compressing data for easier analysis.
Reinforcement Learning:
- Definition: Reinforcement learning involves training an agent to make sequences of decisions in an environment to maximize a reward. The agent learns through trial and error, receiving feedback in the form of rewards or penalties for its actions.
- Example: Teaching an AI agent to play a game where it receives points for making correct moves and loses points for wrong ones. Over time, it learns the best strategies to maximize rewards.
- Applications: Applied in gaming, robotics, autonomous vehicles, and recommendation systems, where systems learn to make sequences of decisions to achieve specific goals or tasks.
Learn About the Impact of Machine Learning
Machine learning stands as a groundbreaking force reshaping industries, ushering in innovation, and reshaping conventional methods. Let's delve into its impact:
"Machine learning is the most influential technology of our era, set to revolutionize every sector."
- Satya Nadella, CEO at Microsoft
Healthcare: In healthcare, machine learning predicts disease outbreaks, tailors patient treatment plans, and enhances medical imaging precision. Google's DeepMind Health collaborates with physicians, employing machine learning to spot diseases earlier, enhancing patient care.
Finance: The finance realm reaps immense benefits from machine learning, employed in credit assessment, algorithmic trading, and fraud detection. A survey shows 56% of global executives integrating AI and machine learning into financial crime compliance programs.
Transportation: Machine learning fuels the self-driving car revolution. Tesla and Waymo utilize machine learning to interpret real-time sensor data, enabling vehicles to identify objects, decide, and navigate autonomously. Moreover, Sweden's Transport Administration collaborates with machine learning specialists to optimize road infrastructure management through computer vision.
Some Machine Learning applications
Machine learning silently powers numerous aspects of our daily lives, driving innovation behind the scenes. Here are some practical examples:
Recommendation Systems
- Companies like Netflix and Amazon employ machine learning to analyze your past actions and suggest products or movies tailored to your preferences. Explore building recommendation engines in Python with our online course.
Voice Assistants
- Siri, Alexa, and Google Assistant utilize machine learning to interpret voice commands and deliver relevant responses. They continuously learn from interactions, enhancing their performance over time.
Fraud Detection
- Banks and credit card firms rely on machine learning to identify fraudulent transactions. By scrutinizing behavioral patterns, they swiftly detect suspicious activities in real time. Dive deeper into fraud detection through our Python course.
Social Media
- Social media platforms leverage machine learning for personalized content curation and content filtering, ensuring tailored experiences while screening inappropriate content.
Machine learning's seamless integration into our daily routines drives efficiency and personalization, making these applications essential components of our modern digital landscape.
You May Also Read: Machine Learning and Deep Learning
Various Tools for Machine Learning:
- TensorFlow: Developed by Google, TensorFlow is an open-source machine learning framework widely used for building and training neural networks across various platforms. It provides extensive libraries and resources for numerical computation, making it popular for deep learning tasks.
- PyTorch: Another popular open-source machine learning library, PyTorch, is particularly favored for its flexibility and ease of use in building neural networks. It's extensively used in research and supports dynamic computation graphs.
- Scikit-learn: This is a user-friendly, open-source machine-learning library in Python. It offers a wide range of tools for traditional machine learning algorithms such as classification, regression, clustering, and more.
- Keras: Often used in conjunction with TensorFlow, Keras is a high-level neural networks API. It simplifies the process of building neural networks and is known for its user-friendly interface.
- Pandas: Pandas is a powerful library in Python for data manipulation and analysis. It provides data structures and tools for handling structured data, which is fundamental in preparing data for machine learning models.
- NumPy: This library is essential for numerical computing in Python. NumPy offers support for large, multi-dimensional arrays and matrices, along with a collection of mathematical functions to operate on these arrays.
- Jupyter Notebooks: Jupyter is a popular open-source web application that allows users to create and share documents containing live code, equations, visualizations, and narrative text. It's widely used for experimentation and prototyping in machine learning projects.
- XGBoost and LightGBM: These are powerful libraries for gradient boosting, often used for building high-performance models in classification and regression tasks.
- Matplotlib and Seaborn: These libraries are used for data visualization in Python, allowing users to create various types of plots and charts to explore and present data insights.
- Spark MLlib: Built on top of Apache Spark, MLlib is a scalable machine learning library designed for distributed computing. It provides a wide range of tools and algorithms for machine learning tasks in big data environments.
Top Machine Learning Careers in 2024
Machine learning has unlocked numerous career paths. From data science to AI engineering, professionals skilled in machine learning are highly sought after. Let's explore these careers:
Data Scientist
Uses scientific methods to extract insights from data. Machine learning is pivotal in predicting and revealing data patterns.
Key Skills:
- Statistical analysis
- Programming (Python, R)
- Machine learning
- Data visualization
- Problem-solving
Essential Tools: Python, R, SQL, Hadoop, Spark, Tableau
Machine Learning Engineer
Designs and implements machine learning systems, experimenting with algorithms and datasets.
Key Skills:
- Programming (Python, Java, R)
- Machine learning algorithms
- Statistics
- System design
Essential Tools: Python, TensorFlow, Scikit-learn, PyTorch, Keras
Research Scientist
Conducts machine learning research, developing new techniques and algorithms.
Key Skills:
- Deep understanding of ML algorithms
- Programming (Python, R)
- Research methodology
- Strong mathematical skills
Essential Tools: Python, R, TensorFlow, PyTorch, MATLAB
How to Begin in Machine Learning Course?
- Grasp Fundamentals:
- Strengthen math and programming basics through online courses.
- Select Tools Wisely:
- Embrace Python along with libraries like NumPy, Pandas, and Scikit-learn.
- Learn Algorithms:
- Progress from simpler to complex ML algorithms. Start with linear regression and expand to neural networks.
- Work on Projects:
- Practice through projects. Start with basic ones like predicting house prices and gradually tackle more complex tasks.
- Stay Updated:
- Keep abreast of ML advancements by following blogs, attending conferences, and engaging in online communities.
Final Thoughts
Machine learning spans multiple industries, fueling innovation. To start, grasp math, programming, and ML algorithms, engage in projects, and stay informed. Whether aiming for data science, AI engineering, or research roles, there's immense potential in this field. Keep learning with leading IT training company like Croma Campus, as ML continually evolves, shaping the future with its transformative capabilities.
Subscribe For Free Demo
Free Demo for Corporate & Online Trainings.
Your email address will not be published. Required fields are marked *
Course Features





