- Nowadays, you get to know in regard of things which can transform out the businesses. When we talk about these factors Data Analyst Online Certification strikes the mind. Resultantly, you think about becoming out Data Analyst which can help out you explore new realms of your career. Moreover, it is important to get out the knowledge of data analytics. As a result of these factors demand for Data Analytics online Training gaining acceleration.
- Most organizations are collecting a huge amount of raw data but in the raw form, data doesnt lead out the meaning of anything. In this scenario, Data Analyst Online Training in India comes into the picture which refers to analyzing raw data in meaningful as well as actionable insights. These insights help in driving efficiency in ongoing businesses. So, the data analyst helps out in the extraction and organizing of the raw data. Hence, Data analyst online certification booming.
- The Data Analyst Online Training offers an overview of Big Data and helps out the candidates in assisting with different complicated procedures. Moreover, emphasizes a variety of Analytics things needed to make our organization efficient. Additionally, Data Analyst Online Training increasing its demand;
By taking up the Data Analyst course in India Understanding Big Data and its applications in the real world.
Analyzing the framework of data structures.
Designing out of complex algorithms.
Implementing Big Data into different activities.
Introducing the candidates with the analytics part.
- After completing the Data Analyst Online course the salary gets out in the range of Rs 1.8 lakh to Rs 11.9 lakh. Moreover, as per the reports of the ambition box, the average salary of candidates post completing the Data Analyst Course India gets struck out at the rate of 15 lakh per annum.
- Getting out of a job in a data analyst course india is the first step in growing out of a career in the domain of Data Analytics. In case you have no previous experience in this domain then you can go through the below-mentioned course prospects after Data Analytics Online Training;
You can become a Data Scientist by advancing your programming skills, advanced mathematics as well as and understanding of machine learning.
Moreover, after completing our Data Analytics Online Training in India the common career path for Data Analytics is to move out to the management process. You can start with the position of Data Analyst, or Project Manager and go to the Chief Data Officer(CDO).
By obtaining a legit data analyst course india, you can easily work in multiple industries. Sometimes, the career path might get deeper into the specialized knowledge of the industry.
After getting out several experiences with completing Data Analytics Online Certification you can work as a consultant in any organization. Moreover, as working out in an organization directly you can also work on a freelance basis.
With a licit certification of data analytics courses in india online, you can also work as a Business analyst, Financial analyst, Operation analyst, Marketing analyst, Systems analyst, etc.
- Undoubtedly, everyone heard about Data Analytics in modern businesses because they are responsible for handling organizational functions. Simply, data analytics is critical to handling organizational projects. As the Data Analytics Online Training gets completed you have to overcome the reasons behind its popularity:
The process of data analytics helps out the decision-makers for improving organizational efficiency. In modern organizations, data analysts are responsible for guiding the business units.
Moreover, after completing our data analyst course online you get out the decisions regarding driving organizational effectiveness, efficiency as well as profitability.
Data analytics is critical to the business point of view that individuals are responsible for determining the effective strategy which drives organizational performance.
As the industry is growing so quickly the demand for related roles is also predicted. Moreover, anyone interested in a strategic business role can get out into this domain.
The job of data analyst requires attention to detail, expert-level statistics, mathematical skills as well and a deep level of understanding of handling organizational processes. And if you wish to turn into a knowledgeable one, obtaining data analytics courses in india online will be a huge boon for your career.
- Data analysts are the professionals who get responsible for the senior leadership in the organization who make out strategic decisions. After completing the Data Analytics Online Training in India you have to follow the below-mentioned primary duties:
Driving out the analysis on unstructured data which comes out from multiple sources.
Building of the NLP models for getting out into key conversation, sentiment as well as emotions.
Working out with multiple projects related to ML(Machine Learning), Text mining.
Analyzing the client-initiated conversations with stakeholders.
Implementing a huge amount of statistical data with text mining.
- After completing the Data Analytics Online course multiple organizations will get to hire out from the pool of candidates. If we talk regarding the organizations then it is Infogain Solutions Pvt Ltd, PubMatic, Serving Skill, Reliance Retail, Huquo Consulting Pvt Ltd, Wipro, CBRE, etc.
- While completing the Data Analytics Online Training in India you get out personalized training with specialized faculty members. Moreover, you also get out 100% globally recognized training certificate which will boost the chances of getting a job in a competitive market.
- Know More About Data Analytics :Click Here
Why should you learn Data Analytics Course?
By registering here, I agree to Croma Campus Terms & Conditions and Privacy Policy
Plenary for Data Analytics Certification Training
Track | Week Days | Weekends | Fast Track |
---|---|---|---|
Course Duration | 40-45 Days | 7 Weekends | 8 Days |
Hours | 1 Hrs. Per Day | 2 Hrs. Per Day | 6+ Hrs. Per Day |
Training Mode | Classroom/Online | Classroom/Online | Classroom/Online |
Want To Know More About
This Course
Program fees are indicative only* Know more
Program Core Credentials

Trainer Profiles
Industry Experts

Trained Students
10000+

Success Ratio
100%

Corporate Training
For India & Abroad

Job Assistance
100%
BATCH TIMINGS
Data Analytics Certification Training Upcoming Batches
WEEKDAY
29-Jul-2024*
Take class during weekdays and utilize your weekend for practice.
Get regular training by Industry Experts.
Get Proper guidance on certifications.
Register for Best Training Program.
10% OFF
FASTRACK
06-Aug-2024*
Running lack of time? Join Fastrack classes to speed up your career growth.
Materials and guidance on certifications
Register for Best Training Program.
WEEKDAY
31-Jul-2024*
Take class during weekdays and utilize your weekend for practice.
Get regular training by Industry Experts.
Get Proper guidance on certifications.
Register for Best Training Program.
10% OFF
WEEKDAY
01-Aug-2024
Take class during weekdays and utilize your weekend for practice.
Get regular training by Industry Experts.
Get Proper guidance on certifications.
Register for Best Training Program.
10% OFF
WEEKEND
27-Jul-2024
More Suitable for working professionals who cannot join in weekdays
Get Intensive coaching in less time
Get Proper guidance on certifications.
Register for Best Training Program.
10% OFF
WEEKEND
10-Aug-2024*
More Suitable for working professionals who cannot join in weekdays
Get Intensive coaching in less time
Get Proper guidance on certifications.
Register for Best Training Program.
10% OFF
Timings Doesn't Suit You ?
We can set up a batch at your convenient time.
Batch Request
FOR QUERIES, FEEDBACK OR ASSISTANCE
Contact Croma Campus Learner Support
Best of support with us
CURRICULUM & PROJECTS
Data Analytics Certification Training
- Data Ananyst is a powerful analytics platform to make discoveries. By using different aspects of computer science, data visualisations, data analytics, statistics, R and Python Programming in data science, you may convert voluminous data into meaningful contents.
- Installation and Working with Python
- Understanding Python variables
- Python basic Operators
- Understanding the Python blocks.
- Python Comments, Multiline Comments.
- Python Indentation
- Understating the concepts of Operators
- Arithmetic
- Relational
- Logical
- Assignment
- Membership
- Identity
- Variables, expression condition and function
- Global and Local Variables in Python
- Packing and Unpacking Arguments
- Type Casting in Python
- Byte objects vs. string in Python
- Variable Scope
- Declaring and using Numeric data types
- Using string data type and string operations
- Understanding Non-numeric data types
- Understanding the concept of Casting and Boolean.
- Strings
- List
- Tuples
- Dictionary
- Sets
- Statements – if, else, elif
- How to use nested IF and Else in Python
- Loops
- Loops and Control Statements.
- Jumping Statements – Break, Continue, pass
- Looping techniques in Python
- How to use Range function in Loop
- Programs for printing Patterns in Python
- How to use if and else with Loop
- Use of Switch Function in Loop
- Elegant way of Python Iteration
- Generator in Python
- How to use nested Loop in Python
- Use If and Else in for and While Loop
- Examples of Looping with Break and Continue Statement
- How to use IN or NOT IN keyword in Python Loop.
- Python Syntax
- Function Call
- Return Statement
- Arguments in a function – Required, Default, Positional, Variable-length
- Write an Empty Function in Python –pass statement.
- Lamda/ Anonymous Function
- *args and **kwargs
- Help function in Python
- Scope and Life Time of Variable in Python Function
- Nested Loop in Python Function
- Recursive Function and Its Advantage and Disadvantage
- Organizing python codes using functions
- Organizing python projects into modules
- Importing own module as well as external modules
- Understanding Packages
- Random functions in python
- Programming using functions, modules & external packages
- Map, Filter and Reduce function with Lambda Function
- More example of Python Function
- Day, Month, Year, Today, Weekday
- IsoWeek day
- Date Time
- Time, Hour, Minute, Sec, Microsec
- Time Delta and UTC
- StrfTime, Now
- Time stamp and Date Format
- Month Calendar
- Itermonthdates
- Lots of Example on Python Calendar
- Create 12-month Calendar
- Strftime
- Strptime
- Format Code list of Data, Time and Cal
- Locale’s appropriate date and time
- What is List.
- List Creation
- List Length
- List Append
- List Insert
- List Remove
- List Append & Extend using “+” and Keyword
- List Delete
- List related Keyword in Python
- List Revers
- List Sorting
- List having Multiple Reference
- String Split to create a List
- List Indexing
- List Slicing
- List count and Looping
- List Comprehension and Nested Comprehension
- What is Tuple
- Tuple Creation
- Accessing Elements in Tuple
- Changing a Tuple
- Tuple Deletion
- Tuple Count
- Tuple Index
- Tuple Membership
- TupleBuilt in Function (Length, Sort)
- Dict Creation
- Dict Access (Accessing Dict Values)
- Dict Get Method
- Dict Add or Modify Elements
- Dict Copy
- Dict From Keys.
- Dict Items
- Dict Keys (Updating, Removing and Iterating)
- Dict Values
- Dict Comprehension
- Default Dictionaries
- Ordered Dictionaries
- Looping Dictionaries
- Dict useful methods (Pop, Pop Item, Str , Update etc.)
- What is Set
- Set Creation
- Add element to a Set
- Remove elements from a Set
- PythonSet Operations
- Frozen Sets
- What is Set
- Set Creation
- Add element to a Set
- Remove elements from a Set
- PythonSet Operations
- Python Errors and Built-in-Exceptions
- Exception handing Try, Except and Finally
- Catching Exceptions in Python
- Catching Specic Exception in Python
- Raising Exception
- Try and Finally
- Opening a File
- Python File Modes
- Closing File
- Writing to a File
- Reading from a File
- Renaming and Deleting Files in Python
- Python Directory and File Management
- List Directories and Files
- Making New Directory
- Changing Directory
- SQL Database connection using
- Creating and searching tables
- Reading and Storing cong information on database
- Programming using database connections
- Installing SMTP Python Module
- Sending Email
- Reading from le and sending emails to all users
- Working With Excel
- Reading an excel le using Python
- Writing to an excel sheet using Python
- Python| Reading an excel le
- Python | Writing an excel le
- Adjusting Rows and Column using Python
- ArithmeticOperation in Excel le.
- Play with Workbook, Sheets and Cells in Excel using Python
- Creating and Removing Sheets
- Formatting the Excel File Data
- More example of Python Function
- Check Dirs. (exist or not)
- How to split path and extension
- How to get user prole detail
- Get the path of Desktop, Documents, Downloads etc.
- Handle the File System Organization using OS
- How to get any les and folder’s details using OS
Introduction To Python
Python Keyword and Identiers
Introduction To Variables
Python Data Type
Control Structure & Flow
Python Function, Modules and Packages
Python Date Time and Calendar
List
Tuple
Dictionary
Sets
Strings
Python Exception Handling
Python File Handling
Python Database Interaction
Contacting user Through Emails Using Python
Reading an excel
Complete Understanding of OS Module of Python
- Data visualization is the graphical way to representation of information and data. By using visual elements like graphs, maps and charts. Data visualization tools provide an accessible easy way to see and understand the data.
- Statistics
- Categorical Data
- Numerical Data
- Mean
- Median
- Mode
- Outliers
- Range
- Interquartile range
- Correlation
- Standard Deviation
- Variance
- Box plot
- Pandas
- Read data from Excel File using Pandas More Plotting, Date Time Indexing and writing to les
- How to get record specic records Using Pandas Adding & Resetting Columns, Mapping with function
- Using the Excel File class to read multiple sheets More Mapping, Filling Nonvalue’s
- Exploring the Data Plotting, Correlations, and Histograms
- Getting statistical information about the data Analysis Concepts, Handle the None Values
- Reading les with no header and skipping records Cumulative Sums and Value Counts, Ranking etc
- Reading a subset of columns Data Maintenance, Adding/Removing Cols and Rows
- Applying formulas on the columns Basic Grouping, Concepts of Aggre gate Function
- Complete Understanding of Pivot Table Data Slicing using iLoc and Loc property (Setting Indices)
- Under sting the Properties of Pivot Table in Pandas Advanced Reading CSVs/HTML, Binning, Categorical Data
- Exporting the results to Excel Joins
- Python | Pandas Data Frame Inner Join
- Under sting the properties of Data Frame Left Join (Left Outer Join)
- Indexing and Selecting Data with Pandas Right Join (Right Outer Join)
- Pandas | Merging, Joining and Concatenating Full Join (Full Outer Join)
- Pandas | Find Missing Data and Fill and Drop NA Appending Data Frame and Data
- Pandas | How to Group Data How to apply Lambda / Function on Data Frame
- Other Very Useful concepts of Pandas in Python Data Time Property in Pandas (More and More)
- NumPy
- Introduction to NumPy Numerical Python
- Importing NumPy and Its Properties
- NumPy Arrays
- Creating an Array from a CSV
- Operations an Array from a CSV
- Operations with NumPy Arrays
- Two-Dimensional Array
- Selecting Elements from 1-D Array
- Selecting Elements from 2-D Array
- Logical Operation with Arrays
- Indexing NumPy elements using conditionals
- NumPy’s Mean and Axis
- NumPy’s Mode, Median and Sum Function
- NumPy’s Sort Function and More
- MatPlotLib
- Bar Chart using Python MatPlotLib
- Column Chart using Python MatPlotLib
- Pie Chart using Python MatPlotLib
- Area Chart using Python MatPlotLib
- Scatter Plot Chart using Python MatPlotLib
- Play with Charts Properties Using MatPlotLib
- Export the Chart as Image
- Understanding plt. subplots () notation
- Legend Alignment of Chart using MatPlotLib
- Create Charts as Image
- Other Useful Properties of Charts.
- Complete Understanding of Histograms
- Plotting Different Charts, Labels, and Labels Alignment etc.
- Introduction to Seaborn
- Introduction to Seaborn
- Making a scatter plot with lists
- Making a count plot with a list
- Using Pandas with seaborn
- Tidy vs Untidy data
- Making a count plot with a Dataframe
- Adding a third variable with hue
- Hue and scattera plots
- Hue and count plots
- Visualizing Two Quantitative Variables
- Introduction to relational plots and subplots
- Creating subplots with col and row
- Customizing scatters plots
- Changing the size of scatter plot points
- Changing the style of scatter plot points
- Introduction to line plots
- Interpreting line plots
- Visualizing standard deviation with line plots
- Plotting subgroups in line plots
- Visualizing a Categorical and a Quantitative Variable
- Current plots and bar plots
- Count plots
- Bar plot with percentages
- Customizing bar plots
- Box plots
- Create and interpret a box plot
- Omitting outliers
- Adjusting the whisk
- Point plots
- Customizing points plots
- Point plot with subgroups
- Customizing Seaborn Plots
- Changing plot style and colour
- Changing style and palette
- Changing the scale
- Using a custom palette
- Adding titles and labels Part 1
- Face Grids vs. Axes Subplots
- Adding a title to a face Grid object
- Adding title and labels Part 2
- Adding a title and axis labels
- Rotating x-tics labels
- Putting it all together
- Box plot with subgroups
- Bar plot with subgroups and subplots
- Well done! What’s next
Data Analysis and Visualization using Pandas.
Data Analysis and Visualization using NumPy and MatPlotLib
Introduction to Data Visualization with Seaborn
- Microsoft SQL Server is a relational database management system (RDBMS) that supports a wide variety of transaction processing, business intelligence and analytics applications in corporate IT environments. In order to experiment with data through the creation of test environments, data scientists make use of SQL as their standard tool, and to carry out data analytics with the data that is stored in relational databases like Oracle, Microsoft SQL, MySQL, we need SQL.
- SQL Server 2019 Installation
- Service Accounts & Use, Authentication Modes & Usage, Instance Congurations
- SQL Server Features & Purpose
- Using Management Studio (SSMS)
- Conguration Tools & SQLCMD
- Conventions & Collation
- SQL Database Architecture
- Database Creation using GUI
- Database Creation using T-SQL scripts
- DB Design using Files and File Groups
- File locations and Size parameters
- Database Structure modications
- SQL Server Database Tables
- Table creation using T-SQL Scripts
- Naming Conventions for Columns
- Single Row and Multi-Row Inserts
- Table Aliases
- Column Aliases & Usage
- Table creation using Schemas
- Basic INSERT
- UPDATE
- DELETE
- SELECT queries and Schemas
- Use of WHERE, IN and BETWEEN
- Variants of SELECT statement
- ORDER BY
- GROUPING
- HAVING
- ROWCOUNT and CUBE Functions
- Table creation using Constraints
- NULL and IDENTITY properties
- UNIQUE KEY Constraint and NOT NULL
- PRIMARY KEY Constraint & Usage
- CHECK and DEFAULT Constraints
- Naming Composite Primary Keys
- Disabling Constraints & Other Options
- Benets of Views in SQL Database
- Views on Tables and Views
- SCHEMA BINDING and ENCRYPTION
- Issues with Views and ALTER TABLE
- Common System Views and Metadata
- Common Dynamic Management views
- Working with JOINS inside views
- Need for Indexes & Usage
- Indexing Table & View Columns
- Index SCAN and SEEK
- INCLUDED Indexes & Usage
- Materializing Views (storage level)
- Composite Indexed Columns & Keys
- Indexes and Table Constraints
- Primary Keys & Non-Clustered Indexes
- Why to use Stored Procedures
- Types of Stored Procedures
- Use of Variables and parameters
- SCHEMABINDING and ENCRYPTION
- INPUT and OUTPUT parameters
- System level Stored Procedures
- Dynamic SQL and parameterization
- Scalar Valued Functions
- Types of Table Valued Functions
- SCHEMABINDING and ENCRYPTION
- System Functions and usage
- Date Functions
- Time Functions
- String and Operational Functions
- ROW_COUNT
- GROUPING Functions
- Why to use Triggers
- DML Triggers and Performance impact
- INSERTED and DELETED memory tables
- Data Audit operations & Sampling
- Database Triggers and Server Triggers
- Bulk Operations with Triggers
- Cursor declaration and Life cycle
- STATIC
- DYNAMIC
- SCROLL Cursors
- FORWARD_ONLY and LOCAL Cursors
- KEYSET Cursors with Complex SPs
- ACID Properties and Scope
- EXPLICIT Transaction types
- IMPLICIT Transactions and options
- AUTOCOMMIT Transaction and usage
- SAVEPOINT and Query Blocking
SQL Server Fundamentals
SQL Server 2019 Database Design
SQL Tables in MS SQL Server
Data Validation and Constraints
Views and Row Data Security
Indexes and Query tuning
Stored Procedures and Benets
System functions and Usage
Triggers, cursors, memory limitations
Cursors and Memory Limitations
Transactions Management
- This module offers knowledge to introduce you to the basic principles based on statistical methods and procedures followed in data analysis. This course will help you to understand the work process involved with summarizing the data, data storage, visualizing the data results, and a hands-on approach with statistical analysis with python.
- What is Analytics & Data Science
- Common Terms in Data Science
- What is data
- Classication of data
- Relevance in industry and need of the hour
- Types of problems and business objectives in various industries
- How leading companies are harnessing the power of analytics
- Critical success drivers.
- Overview of Data Science tools & their popularity.
- Data Science Methodology & problem-solving framework.
- List of steps in Data Science projects
- Identify the most appropriate solution design for the given problem statement
- Project plan for Data Science project & key milestones based on effort estimates
- Build Resource plan for Data Science project
- Why Python for data science
- Importing Data from various sources (Csv, txt, excel, access etc)
- Database Input (Connecting to database)
- Viewing Data objects - sub setting, methods
- Exporting Data to various formats
- Important python modules Pandas
- Cleansing Data with Python
- Filling missing values using lambda function and concept of Skewness.
- Data Manipulation steps (Sorting, ltering, duplicates, merging, append ing, sub setting, derived variables, sampling, Data type conversions, renaming, formatting.
- Normalizing data
- Feature Engineering
- Feature Selection
- Feature scaling using Standard Scaler/Min-Max scaler/Robust Scaler.
- Label encoding/one hot encoding
- Introduction exploratory data analysis
- Descriptive statistics, Frequency Tables and summarization
- Univariate Analysis (Distribution of data & Graphical Analysis)
- Bivariate Analysis (Cross Tabs, Distributions & Relationships, Graphical Analysis)
- Creating Graphs- Bar/pie/line chart/histogram/ boxplot/ scatter/ densi ty etc.)
- Important Packages for Exploratory Analysis (NumPy Arrays, Matplotlib, seaborn, Pandas etc.)
- Descriptive Statistics
- Sample vs Population Statistics
- Random variables
- Probability distribution functions
- Expected value
- Normal distribution
- Gaussian distribution
- Z-score
- Spread and Dispersion
- Correlation and Co-variance
- Concept of model in analytics and how it is used
- Common terminology used in Analytics & Modelling process
- Popular Modelling algorithms
- Types of Business problems - Mapping of Techniques
- Different Phases of Predictive Modelling
- Need for structured exploratory data
- EDA framework for exploring the data and identifying any problems with the data (Data Audit Report)
- Identify missing data
- Identify outliers’ data
- Imbalanced Data Techniques
- Data Preparation
- Feature Engineering
- Feature Scaling
- Datasets
- Dimensionality Reduction
- Anomaly Detection
- Parameter Estimation
- Data and Knowledge
- Selected Applications in Data Mining
Introduction to Data Analytics
Accessing/Importing and Exporting Data
Data Manipulation Cleansing - Munging Using Python Modules
Feature Engineering in Data Science
Data Analysis Visualization Using Python
Introduction to Statistics
Introduction to Predictive Modelling
EDA (Exploratory Data Analysis)
Data Pre-Processing & Data Mining
- Excel is one of the most popular data analysis tool, to help visualize and gain insights from your data. Analytics with Excel helps you to boost your Microsoft Excel skills.
- Creation of Excel Sheet Data
- Range Name, Format Painter
- Conditional Formatting, Wrap Text, Merge & Centre
- Sort, Filter, Advance Filter
- Different type of Chart Creations
- Auditing, (Trace Precedents, Trace Dependents)Print Area
- Data Validations, Consolidate, Subtotal
- What if Analysis (Data Table, Goal Seek, Scenario)
- Solver, Freeze Panes
- Various Simple Functions in Excel(Sum, Average, Max, Min)
- Real Life Assignment work
- Advance Data Sorting
- Multi-level sorting
- Restoring data to original order after performing sorting
- Sort by icons
- Sort by colours
- Lookup Functions
- Lookup
- VLookup
- HLookup
- Subtotal, Multi-Level Subtotal
- Grouping Features
- Column Wise
- Row Wise
- Consolidation With Several Worksheets
- Filter
- Auto Filter
- Advance Filter
- Printing of Raw & Column Heading on Each Page
- Workbook Protection and Worksheet Protection
- Specified Range Protection in Worksheet
- Excel Data Analysis
- Goal Seek
- Scenario Manager
- Data Table
- Advance use of Data Tables in Excel
- Reporting and Information Representation
- Pivot Table
- Pivot Chat
- Slicer with Pivot Table & Chart
- Generating MIS Report In Excel
- Advance Functions of Excel
- Math & Trig Functions
- Text Functions
- Lookup & Reference Function
- Logical Functions & Date and Time Functions
- Database Functions
- Statistical Functions
- Financial Functions
- Functions for Calculation Depreciation
- Dashboard Background
- Dashboard Elements
- Interactive Dashboards
- Type of Reporting In India
- Reporting Analyst
- Indian Print Media Reporting
- Audit Report
- Accounting MIS Reports
- HR Mis Reports
- MIS Report Preparation Supplier, Exporter
- Data Analysis
- Costing Budgeting Mis Reporting
- MIS Report For Manufacturing Company
- MIS Reporting For Store And Billing
- Product Performance Report
- Member Performance Report
- Customer-Wise Sales Report
- Collections Report
- Channel Stock Report
- Prospect Analysis Report
- Calling Reports
- Expenses Report
- Stock Controller MIS Reporting
- Inventory Statement
- Payroll Report
- Salary Slip
- Loan Assumption Sheet
- Invoice Creation
Understanding Concepts of Excel
Ms Excel Advance
MIS Reporting & Dash Board
- The Power BI course assists the user to understand the way to install Power BI desktop also by understanding and developing the workshop and insights using the data. It offers tools and techniques that are used to visualize and analyze data. The course will help you to learn and grab insights on everything an organization need; to manage the data with Power BI.
- Overview of BI concepts
- Why we need BI
- Introduction to SSBI
- SSBI Tools
- Why Power BI
- What is Power BI
- Building Blocks of Power BI
- Getting started with Power BI Desktop
- Get Power BI Tools
- Introduction to Tools and Terminology
- Dashboard in Minutes
- Interacting with your Dashboards
- Sharing Dashboards and Reports
- Power BI Desktop
- Extracting data from various sources
- Workspaces in Power BI
- Data Transformation
- Query Editor
- Connecting Power BI Desktop to our Data Sources
- Editing Rows
- Understanding Append Queries
- Editing Columns
- Replacing Values
- Formatting Data
- Pivoting and Unpivoting Columns
- Splitting Columns
- Creating a New Group for our Queries
- Introducing the Star Schema
- Duplicating and Referencing Queries
- Creating the Dimension Tables
- Entering Data Manually
- Merging Queries
- Finishing the Dimension Table
- Introducing the another DimensionTable
- Creating an Index Column
- Duplicating Columns and Extracting Information
- Creating Conditional Columns
- Creating the FACT Table
- Performing Basic Mathematical Operations
- Improving Performance and Loading Data into the Data Model
- Introduction to Modelling
- Modelling Data
- Manage Data Relationship
- Optimize Data Models
- Cardinality and Cross Filtering
- Default Summarization & Sort by
- Creating Calculated Columns
- Creating Measures & Quick Measures
- What is DAX
- Data Types in DAX
- Calculation Types
- Syntax, Functions, Context Options
- DAX Functions
- Date and Time
- Time Intelligence
- Information
- Logical
- Mathematical
- Statistical
- Text and Aggregate
- Measures in DAX
- Measures and Calculated Columns
- ROW Context and Filter Context in DAX
- Operators in DAX - Real-time Usage
- Quick Measures in DAX - Auto validations
- In-Memory Processing DAX Performance
- How to use Visual in Power BI
- What Are Custom Visuals
- Creating Visualisations and Colour Formatting
- Setting Sort Order
- Scatter & Bubble Charts & Play Axis
- Tooltips and Slicers, Timeline Slicers & Sync Slicers
- Cross Filtering and Highlighting
- Visual, Page and Report Level Filters
- Drill Down/Up
- Hierarchies and Reference/Constant Lines
- Tables, Matrices & Conditional Formatting
- KPI's, Cards & Gauges
- Map Visualizations
- Custom Visuals
- Managing and Arranging
- Drill through and Custom Report Themes
- Grouping and Binning and Selection Pane, Bookmarks & Buttons
- Data Binding and Power BI Report Server
- Why Dashboard and Dashboard vs Reports
- Creating Dashboards
- Conguring a Dashboard Dashboard Tiles, Pinning Tiles
- Power BI Q&A
- Quick Insights in Power BI
- Custom Data Gateways
- Exploring live connections to data with Power BI
- Connecting directly to SQL Server
- Connectivity with CSV & Text Files
- Excel with Power BI Connect Excel to Power BI, Power BI Publisher for Excel
- Content packs
- Update content packs
- Introduction and Sharing Options Overview
- Publish from Power BI Desktop and Publish to Web
- Share Dashboard with Power BI Service
- Workspaces (Power BI Pro) and Content Packs (Power BI Pro)
- Print or Save as PDF and Row Level Security (Power BI Pro)
- Export Data from a Visualization
- Export to PowerPoint and Sharing Options Summary
- Understanding Data Refresh
- Personal Gateway (Power BI Pro and 64-bit Windows)
- Replacing a Dataset and Troubleshooting Refreshing
Introduction to Power BI
Power BI Desktop
Power BI Data Transformation
Modelling with Power BI
Data Analysis Expressions (DAX)
Power BI Desktop Visualisations
Introduction to Power BI Dashboard and Data Insights
Direct Connectivity
Publishing and Sharing
Refreshing Datasets
- Tableau is an end-to-end data analytics platform that allows you to prep, analyze, collaborate, and share your data insights. Tableau excels in self-service visual analysis, allowing people to ask new questions of governed big data and easily share those insights across the organization.
- Data Visualization
- Business Intelligence tools
- Introduction to Tableau
- Tableau Architecture
- Tableau Server Architecture
- VizQL Fundamentals
- Introduction to Tableau Prep
- Tableau Prep Builder User Interface
- Data Preparation techniques using Tableau Prep Builder tool
- Features of Tableau Desktop
- Connect to data from File and Database
- Types of Connections
- Joins and Unions
- Data Blending
- Tableau Desktop User Interface
- Visual Analytics
- Basic Charts Bar Chart, Line Chart, and Pie Chart
- Hierarchies
- Data Granularity
- Highlighting
- Sorting
- Filtering
- Grouping
- Sets
- Types of Calculations
- Built-in Functions (Number, String, Date, Logical and Aggregate)
- Operators and Syntax Conventions
- Table Calculations
- Level of Detail (LOD) Calculations
- Using R within Tableau for Calculations
- Parameters
- Tool tips
- Trend lines
- Reference lines
- Forecasting
- Clustering
- Count Customer by Order
- Profit per Business Day
- Comparative Sales
- Profit Vs Target
- Finding the second order date
- Cohort Analysis
- Introduction to Geographic Visualizations
- Manually assigning Geographical Locations
- Types of Maps
- Spatial Files
- Custom Geocoding
- Polygon Maps
- Web Map Services
- Background Images
- Box and Whisker’s Plot
- Bullet Chart
- Bar in Bar Chart
- Gantt Chart
- Waterfall Chart
- Pareto Chart
- Control Chart
- Funnel Chart
- Bump Chart
- Step and Jump Lines
- Word Cloud
- Donut Chart
- Introduction to Dashboards
- The Dashboard Interface
- Dashboard Objects
- Building a Dashboard
- Dashboard Layouts and Formatting
- Interactive Dashboards with actions
- Designing Dashboards for devices
- Story Points
- Tableau Tips and Tricks
- Choosing the right type of Chart
- Format Style
- Data Visualization best practices
- Publishing Workbooks to Tableau Online
- Interacting with Content on Tableau Online
- Data Management through Tableau Catalog
- AI-Powered features in Tableau Online (Ask Data and Explain Data)
- Understand Scheduling
- Managing Permissions on Tableau Online
- Data Security with Filters in Tableau Online
Introduction to Data Preparation using Tableau Prep
Data Connection with Tableau Desktop
Basic Visual Analytics
Calculations in Tableau
Advanced Visual Analytics
Level of Detail (LOD) Expressions in Tableau
Geographic Visualizations in Tableau
Advanced charts in Tableau
Dashboards and Stories
Get Industry Ready
Exploring Tableau Online
- AWS allows you to easily move data between the data lake and purpose-built data services. For example, AWS Glue is a serverless data integration service that makes it easy to prepare data for analytics, machine learning, and application development.
- In this module, you will learn what Cloud Computing is and what are the different models of Cloud Computing along with the key differentiators of different models. We will also introduce you to virtual world of AWS along with AWS key vocabulary, services and concepts.
- A Short history
- Client Server Computing Concepts
- Challenges with Distributed Computing
- Introduction to Cloud Computing
- Why Cloud Computing
- Benefits of Cloud Computing
- In this module, you will learn about the introduction to compute offering from AWS called EC2. We will cover different instance types and Amazon AMIs. A demo on launching an AWS EC2 instance, connect with an instance and host ing a website on AWS EC2 instance. We will also cover EBS storage Architecture (AWS persistent storage) and the concepts of AMI and snapshots.
- Amazon EC2
- EC2 Pricing
- EC2 Type
- Installation of Web server and manage like (Apache/ Nginx)
- Demo of AMI Creation
- Exercise
- Hands on both Linux and Windows
- In this module, you will learn how AWS provides various kinds of scalable storage services. In this module, we will cover different storage services like S3, Glacier, Versioning, and learn how to host a static website on AWS.
- Versioning
- Static website
- Policy
- Permission
- Cross region Replication
- AWS-CLI
- Life cycle
- Classes of Storage
- AWS CloudFront
- Real scenario Practical
- Hands-on all above
- In this module, you will learn how to monitoring AWS resources and setting up alerts and notifications for AWS resources and AWS usage billing with AWS CloudWatch and SNS.
- Amazon Cloud Watch
- SNS - Simple Notification Services
- Cloud Watch with Agent
- In this module, you will learn about 'Scaling' and 'Load distribution techniques' in AWS. This module also includes a demo of Load distribution & Scaling your resources horizontally based on time or activity.
- Amazon Auto Scaling
- Auto scaling policy with real scenario based
- Type of Load Balancer
- Hands on with scenario based
- In this module, you will learn introduction to Amazon Virtual Private Cloud. We will cover how you can make public and private subnet with AWS VPC. A demo on creating VPC. We will also cover overview of AWS Route 53.
- Amazon VPC with subnets
- Gateways
- Route Tables
- Subnet
- Cross region Peering
- In this module, you will learn how to achieve distribution of access control with AWS using IAM.
- Amazon IAM
- add users to groups,
- manage passwords,
- log in with IAM-created users.
- User
- Group
- Role
- Policy
- In this module, you will learn how to manage relational database service of AWS called RDS.
- Amazon RDS
- Type of RDS
- RDS Failover
- RDS Subnet
- RDS Migration
- Dynamo DB (No SQL DB)
- Redshift Cluster
- SQL workbench
- JDBC / ODBC
- In this module, you will get an overview of multiple AWS services. We will talk about how do you manage life cycle of AWS resources and follow the DevOps model in AWS. We will also talk about notification and email service of AWS along with Content Distribution Service in this module.
- Cloud Trail,
- In this module, you will cover various architecture and design aspects of AWS. We will also cover the cost planning and optimization techniques along with AWS security best practices, High Availability (HA) and Disaster Recovery (DR) in AWS.
- AWS High Availability Design
- AWS Best Practices (Cost +Security)
- AWS Calculator & Consolidated Billing
- Public DNS
- Private DNS
- Routing policy
- Records
- Register DNS
- Work with third party DNS as well
- Stack
- Templet
- Json / Ymal
- Installation of Linux
- Configuration
- Manage
- Installation of app on Linux (apache / Nginx etc)
- AWS cli configuration on Linux
- Complete hands-on on Linux.
- Scenario based lab and practical
- Each topic and services will be cover with lab and theory.
Introduction to Cloud Computing
Amazon EC2 and Amazon EBS
Amazon Storage Services S3 (Simple Storage Services)
Cloud Watch & SNS
Scaling and Load Distribution in AWS
AWS VPC
Identity and Access Management Techniques (IAM)
Amazon Relational Database Service (RDS)
Multiple AWS Services and Managing the Resources' Lifecycle
AWS Architecture and Design
Migrating to Cloud & AWS
Router S3 DNS
Cloud Formation
Elastic Beanstalk
EFS / NFS (hands-on practice)
Hands-on practice on various Topics
Linux
- Data Scientists know how to train Predictive Models. So, by enabling them to work together, Microsoft Azure Data Science ensures high-quality models at scale in production. With MLOps incorporated as a part of the Microsoft Azure Data Science platform, Data Scientists can create a discrete pipeline for each model
- Identify the benefits and considerations of using cloud services Cloud Computing Basics.
- Identify the benefits of cloud computing, such as High Availability,Scalability, Elasticity,
- Agility, and Disaster Recovery
- Identify the differences between Capital Expenditure (Cap Ex) and Operational.
- Expenditure (Op Ex)
- Describe the consumption-based model
- Describe the differences between categories of cloud services
- Describe the shared responsibility model
- Describe Infrastructure-as-a-Service (IaaS),
- Describe Platform-as-a-Service (PaaS)
- Describe server less computing
- Describe Software-as-a-Service (SaaS)
- Identify a service type based on a use case
- Describe the differences between types of cloud computing
- Define cloud computing
- Describe Public cloud
- Describe Private cloud
- Describe Hybrid cloud
- Compare and contrast the three types of cloud computing Describe Core Azure Services
- Manage Azure AD objects
- create users and groups
- manage user and group properties
- manage device settings
- perform bulk user updates
- manage guest accounts
- configure Azure AD Join
- configure self-service password reset
- NOTE Azure AD Connect; PIM
- Manage role-based access control (RBAC)
- create a custom role
- provide access to Azure resources by assigning roles
- subscriptions
- resource groups
- resources (VM, disk, etc.)
- interpret access assignments
- manage multiple directories
- Manage subscriptions and governance
- configure Azure policies
- configure resource locks
- apply tags
- create and manage resource groups
- move resources
- remove RGs
- manage subscriptions
- configure Cost Management
- configure management groups
- Manage storage accounts
- configure network access to storage accounts
- create and configure storage accounts
- generate shared access signature
- manage access keys
- implement Azure storage replication
- configure Azure AD Authentication for a storage account
- Manage data in Azure Storage
- export from Azure job
- import into Azure job
- install and use Azure Storage Explorer
- copy data by using AZ Copy
- Configure Azure files and Azure blob storage
- create an Azure file share
- create and configure Azure File Sync service
- configure Azure blob storage
- configure storage tiers for Azure blobs
- Configure VMs for high availability and scalability
- configure high availability
- deploy and configure scale sets
- Create and configure VMs
- configure Azure Disk Encryption
- move VMs from one resource group to another
- manage VM sizes
- add data discs
- configure networking
- redeploy VMs
- Create and configure Web Apps
- create and configure App Service
- create and configure App Service Plans
- Implement and manage virtual networking
- create and configure VNET peering
- configure private and public IP addresses, network routes, network interface,
- subnets, and virtual network
- Configure name resolution
- configure Azure DNS
- configure custom DNS settings
- configure a private or public DNS zone
- Secure access to virtual networks
- create security rules
- associate an NSG to a subnet or network interface
- evaluate effective security rules
- deploy and configure Azure Firewall
- deploy and configure Azure Bastion Service
- NOT Implement Application Security Groups; DDoS
- Configure load balancing
- configure Application Gateway
- configure an internal load balancer
- configure load balancing rules
- configure a public load balancer
- troubleshoot load balancing
- NOT Traffic Manager and Front Door and Private Link
- Implement backup and recovery
- configure and review backup reports
- perform backup and restore operations by using Azure Backup Service
- create a Recovery Services Vault
- use soft deletes to recover Azure VMs
Describe Cloud Concepts
Manage Azure identities and governance (15-20%)
Implement and Manage Storage (10-15%)
Deploy and Manage Azure Compute Resources (25-30%)
Configure and Manage Virtual Networking (30-35%)
Monitor and Back up Azure Resources (10-15%)
- The training offers complete career transitioning projects based on the current needs of the organization. These projects are guided by experts and help you to add more value to your profile. You will learn to initiate data analytics projects based on a high-level perspective helping you to understand and articulate the innovative solutions.
- Managing credit card Risks
- Bank Loan default classification
- YouTube Viewers prediction
- Super store Analytics (E-commerce)
- Buying and selling cars prediction (like OLX process)
- Advanced House price prediction
- Analytics on HR decisions
- Survival of the fittest
- Twitter Analysis
- Flight price prediction
Here is the project list you will going to work on
+ More Lessons
Mock Interviews

Projects
Phone (For Voice Call):
+91-971 152 6942WhatsApp (For Call & Chat):
+918287060032self assessment
Learn, Grow & Test your skill with Online Assessment Exam to achieve your Certification Goals
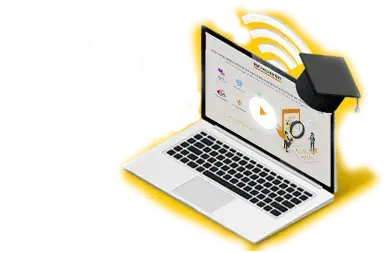
FAQ's
Croma campus is one of the best institute for training of IT professional jobs. It is one of the most prestigious and certified organizations that has been associated with the top most MNCs. Croma campus is situated in Noida which is really famous for its innovative and technical teaching methods. So, if you want to get linked with Data Analytics then do a Collab with Croma Campus.
Data Analytics is a process of examining datasets to know about the information they contain. There are a lot of works and jobs under Data Analytics. The first thing you need to do is to always keep your profile updated on LinkedIn because they are directly associated with Data Analytics. So, if you get an Data Analytics certificate you can work as Data Analyst.
Data Analytics is nowadays becoming a very important certification that can lead you to get a good job. To get any Data Analyst job you need to get a certification in Data Analytics. There is a list of things after which you can get a certificate that contains in-depth training, many simultaneously exams, live demos, and other industrial projects that can make you a perfect Data Analyst. After all this training, you can get an Data Analytics certification.
Croma Campus India program sizes a powerful training tool that can be applied in classrooms as well as in manufacturing. We offer a wide range of agendas for Live Project Data Analytics Training in India under the leadership of the best industrial experts. We are always awarded for the past 10 years as the Best Data Analytics Online Training in India.
The ways to connect Croma Campus
- Phone Number: - +91-120-4155255, +91-9711526942
- Email: - info@cromacampus.com
- Address: - G-21, Sector-03, Noida (201301)
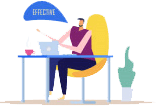
- - Build an Impressive Resume
- - Get Tips from Trainer to Clear Interviews
- - Attend Mock-Up Interviews with Experts
- - Get Interviews & Get Hired
If yes, Register today and get impeccable Learning Solutions!
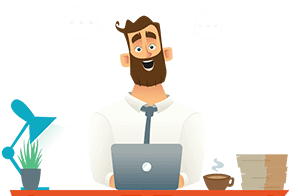
Training Features
Instructor-led Sessions
The most traditional way to learn with increased visibility,monitoring and control over learners with ease to learn at any time from internet-connected devices.
Real-life Case Studies
Case studies based on top industry frameworks help you to relate your learning with real-time based industry solutions.
Assignment
Adding the scope of improvement and fostering the analytical abilities and skills through the perfect piece of academic work.
Lifetime Access
Get Unlimited access of the course throughout the life providing the freedom to learn at your own pace.
24 x 7 Expert Support
With no limits to learn and in-depth vision from all-time available support to resolve all your queries related to the course.

Certification
Each certification associated with the program is affiliated with the top universities providing edge to gain epitome in the course.
Training Certification
Your certificate and skills are vital to the extent of jump-starting your career and giving you a chance to compete in a global space.
Talk about it on Linkedin, Twitter, Facebook, boost your resume or frame it- tell your friend and colleagues about it.
Video Reviews
Testimonials & Reviews
I was enrolled for the R programming under the guidance of Mr. Anantha Rao sir. He has a very unique way of teaching and daily presentations by the students were the main highlight of the class. Whatever he taught is actually really he
Read More...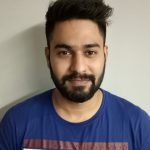
Tinish Sharma
TCS R programming
Best training institute for learning Tableau. Anurag Mishra sir is the best trainer for learning Tableau.
Read More...Ishani
Data Analytics
Hi. I have done this course Croma campus. The trainer give the sessions very nicely nd in-depth knowledge. My experience is good throughout the learning program. Management is good. The course helped me in increasing my knowledge and c
Read More...Aadesh
Data Analytics
If you are looking to get a reputed job after SAS certification then you should go for the top which is Croma Campus. I have recently completed my SAS certification and got a placement in a reputed MNC. Moreover, the training offered o
Read More...Aakash
Data Analytics
Hi, my name is Anup Singh and I have recently completed my SAS certification from Croma Campus. I am fully satisfied after getting a world-class experience at affordable costs. Besides offering out the training they also guided me rega
Read More...Anup Singh
Data Analytics
Hi, my name is Manoj Diwakar I have recently completed our Microsoft Excel VBA Certification from Croma Campus Pvt Ltd. It helps out me in clearing the doubts related to training and prepares me for further job interviews.
Read More...Manoj Diwakar
Data Analytics
Croma Campus is a very nice training center. I have taken SPSS training in Croma Campus. They are very experienced and the way of teaching is very good. I got the job based on training; it was my great experience at Croma Campus. All t
Read More...Aanav
Data Analytics
Hi, my name is Neelam. I currently work as a Microsoft Excel at HCL. My interview in this company was scheduled by Croma Campus as I have done 2 months of Microsoft Advance Excel Certification Training course from it. I managed to crac
Read More...Neelam
Currently working at RBL .The knowledge and the environment which I got in the croma campus was very helpful to achieve the success.
Read More...Upasana Singh